When training AI software, the choice of video source plays a crucial role in determining the effectiveness and accuracy of the training process. The best fit for training AI software would be high-quality, diverse video sources that provide a wide range of visual data for the AI to learn from.
These sources should include a variety of scenarios, environments, and objects to ensure that the AI is exposed to a comprehensive set of data. Additionally, the video sources should be well-labeled and annotated to provide context and meaning to the AI, helping it to better understand and interpret the visual information.
Furthermore, the video sources should be up-to-date and relevant to the specific task or application that the AI software is being trained for.
By using high-quality, diverse, well-labeled, and relevant video sources, AI software can be trained more effectively and accurately, leading to better performance and results in real-world applications.
What types of video sources are ideal for training ai software?
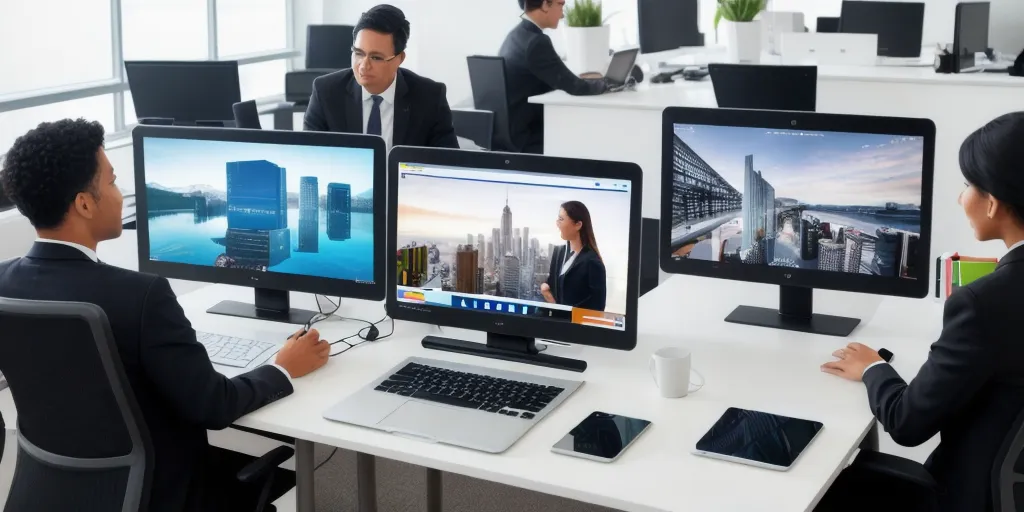
When training AI software, it is essential to use a variety of video sources to ensure comprehensive learning and accurate results. Ideal video sources for training AI software include high-quality videos with clear visuals and audio, diverse content that covers a wide range of topics and scenarios, and a large dataset to provide ample examples for the AI to learn from.
Additionally, videos that are well-labeled and categorized can help the AI software better understand and interpret the information it is being exposed to.
Real-world videos that reflect common situations and interactions can also be beneficial in training AI software to recognize and respond to various scenarios accurately. Furthermore, incorporating videos with different perspectives, languages, and cultural contexts can help AI software develop a more robust understanding of the world around it.
By utilizing a diverse range of video sources in training AI software, developers can ensure that the AI is well-equipped to handle a wide array of tasks and challenges effectively.
How important is the quality of video sources in training ai software?
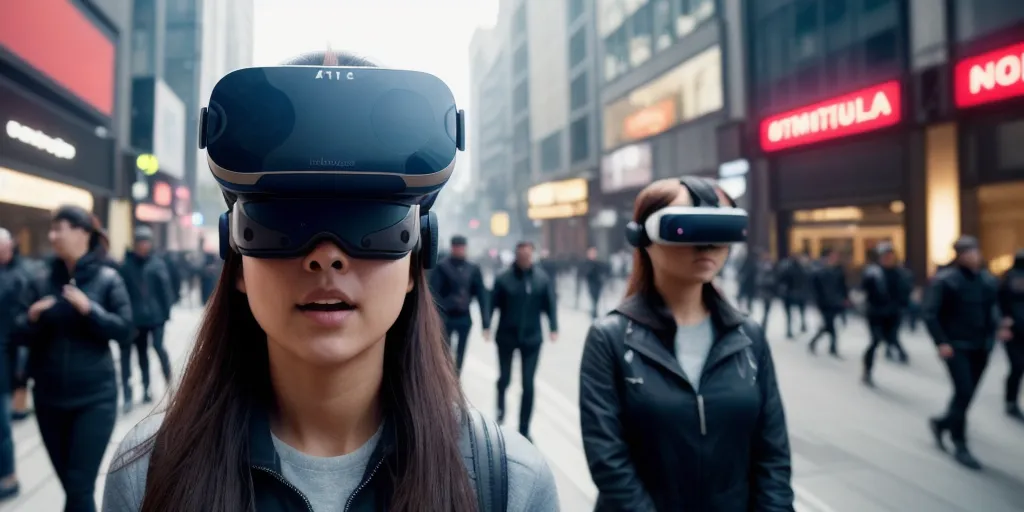
The quality of video sources plays a crucial role in training AI software. High-quality video sources provide clear and detailed information that is essential for AI algorithms to accurately learn and make predictions. Poor-quality video sources, on the other hand, can lead to inaccurate or incomplete data, which can negatively impact the performance of AI software.
When training AI models, it is important to use high-resolution videos with good lighting and minimal noise to ensure that the algorithms can effectively analyze and interpret the visual data.
Additionally, the quality of video sources can also affect the speed and efficiency of training AI software. Low-quality videos may require more processing power and time to train the algorithms, leading to increased costs and longer development cycles.
Therefore, investing in high-quality video sources is essential for achieving optimal results when training AI software and ensuring that the algorithms can accurately understand and interpret visual information. Ultimately, the quality of video sources directly impacts the performance and effectiveness of AI software, making it a critical factor to consider in the development and training process.
Why is it crucial for video sources to be diverse when training ai software?
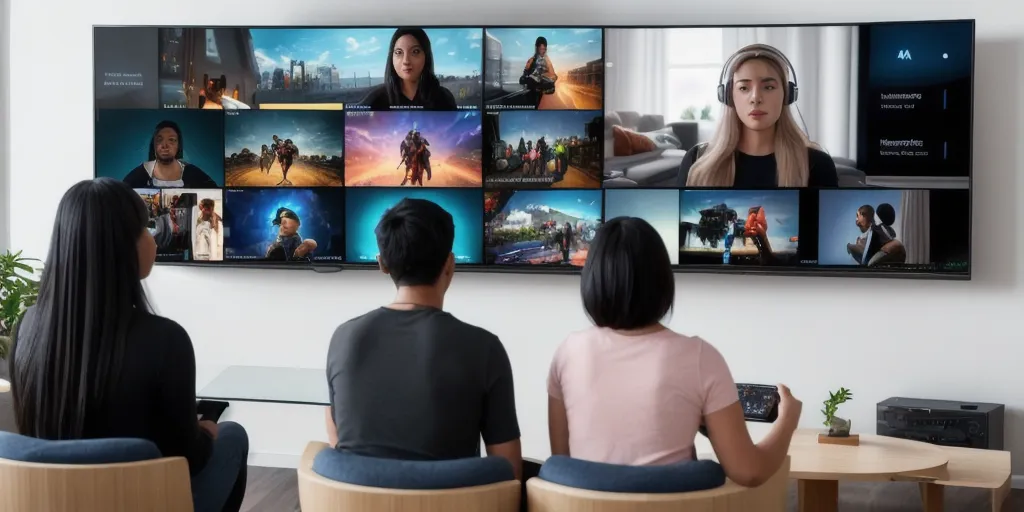
It is crucial for video sources to be diverse when training AI software for several reasons. Firstly, a diverse range of video sources helps to ensure that the AI software is exposed to a wide variety of scenarios, environments, and perspectives.
This exposure allows the AI to learn more effectively and accurately, as it can draw upon a broader range of experiences and information. Additionally, using diverse video sources helps to prevent bias in the AI’s training data. If the AI is only trained on a narrow set of video sources, it may develop biases or limitations that could impact its performance and decision-making abilities.
By incorporating a diverse range of video sources, developers can help to create more inclusive and unbiased AI systems that are better equipped to handle a wide range of tasks and challenges.
Overall, diversity in video sources is essential for training AI software to be more versatile, accurate, and fair in its decision-making processes.
How do well-labeled and annotated video sources benefit the training of ai software?
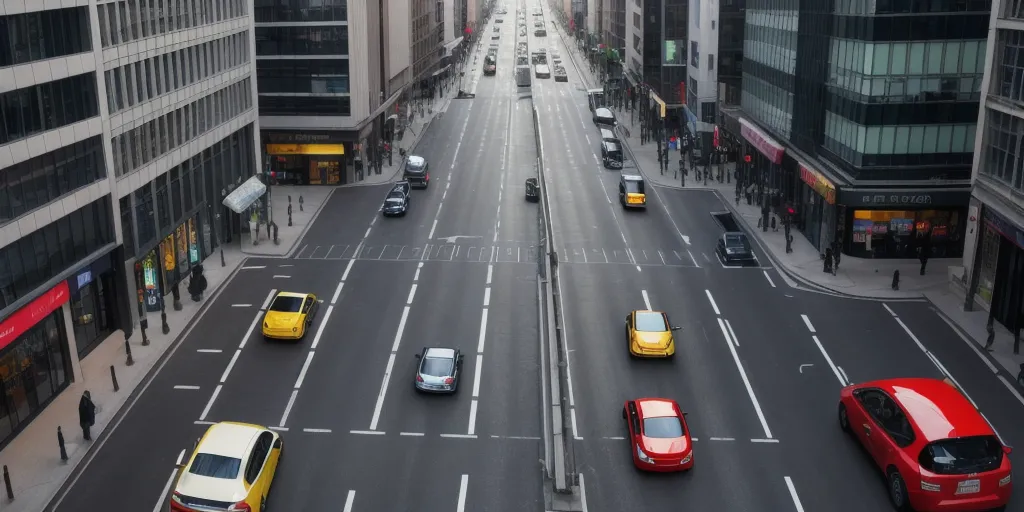
Well-labeled and annotated video sources play a crucial role in enhancing the training of AI software in various ways. Firstly, these sources provide a structured and organized dataset for the AI algorithms to learn from, enabling them to recognize patterns and make accurate predictions.
By labeling different objects, actions, and scenarios within the videos, the AI software can better understand the context and meaning of the visual information it processes. This leads to more precise and reliable outcomes when the software is deployed for tasks such as object recognition, video analysis, and autonomous driving.
Additionally, annotated video sources help in reducing the time and effort required for training AI models, as the labeled data accelerates the learning process and improves the overall efficiency of the system.
Moreover, well-labeled videos enable researchers and developers to track the performance and progress of the AI software, allowing them to identify areas for improvement and fine-tune the algorithms accordingly. In conclusion, well-labeled and annotated video sources are essential for enhancing the training of AI software by providing a solid foundation of labeled data, improving accuracy and efficiency, and facilitating continuous optimization of the algorithms.