To upscale video using AI, you can utilize various deep learning algorithms and neural networks that are specifically designed for enhancing video quality. One popular method is using convolutional neural networks (CNNs) to upscale low-resolution videos to higher resolutions.
By training the AI model on a dataset of high-resolution videos, the network can learn to predict missing pixels and enhance the overall quality of the video. Another approach is to use generative adversarial networks (GANs) to generate realistic high-resolution images from low-resolution inputs.
GANs work by training two neural networks simultaneously – a generator network that creates high-resolution images and a discriminator network that evaluates the generated images for realism. By iteratively improving the generator network based on feedback from the discriminator, GANs can produce high-quality upscaled videos.
Additionally, there are AI-powered video upscaling software tools available that can automatically enhance the resolution, sharpness, and overall quality of videos using advanced algorithms. By leveraging AI technology, you can easily upscale videos to achieve better visual quality and clarity.
How can ai enhance video quality?
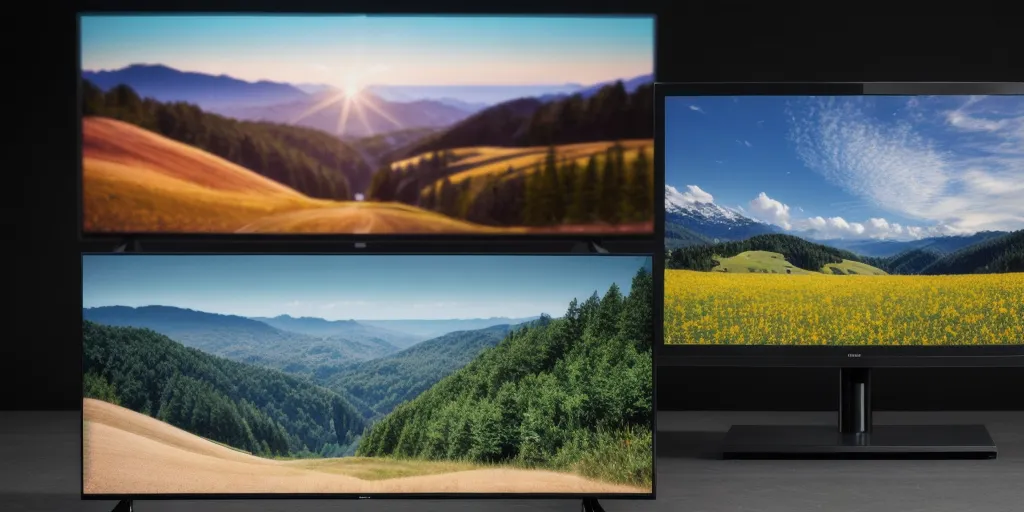
Artificial Intelligence (AI) can enhance video quality in several ways. One of the main ways AI can improve video quality is through the use of upscaling techniques. AI algorithms can analyze low-resolution videos and intelligently predict missing pixels to enhance the overall quality of the video.
This can result in sharper images and clearer details, making the video more visually appealing. Additionally, AI can also be used to reduce noise and artifacts in videos, resulting in a cleaner and more professional-looking final product.
Another way AI can enhance video quality is through the use of image recognition technology. AI algorithms can analyze video content in real-time and make adjustments to color, lighting, and contrast to improve overall visual quality. This can help to create more vibrant and engaging videos that capture the viewer’s attention.
Overall, AI has the potential to revolutionize the way we create and consume video content by enhancing quality and improving the overall viewing experience.
What neural networks are used for upscaling videos?
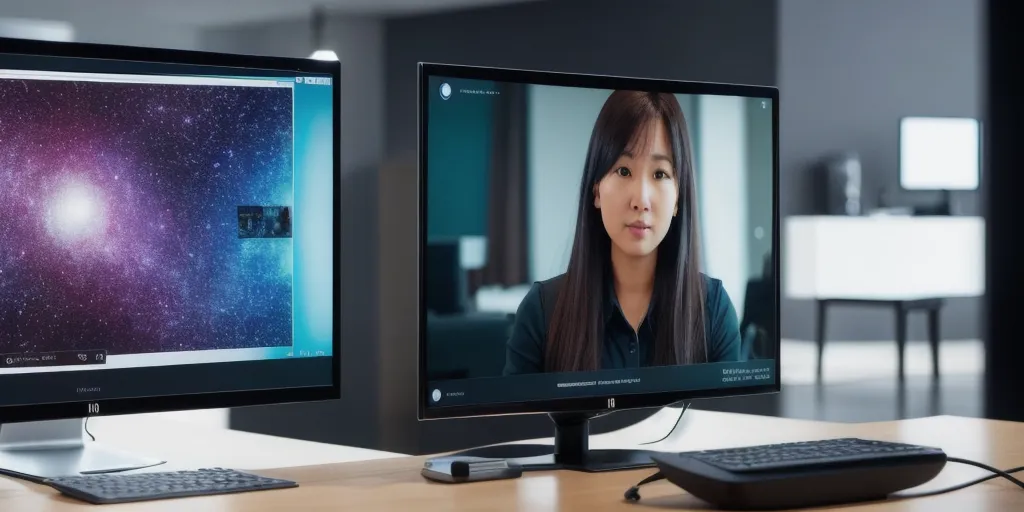
Neural networks are commonly used for upscaling videos to enhance their resolution and quality. One of the most popular neural networks used for this purpose is the Generative Adversarial Network (GAN). GANs consist of two neural networks – a generator and a discriminator – that work together to produce high-resolution images.
The generator creates new images based on the input data, while the discriminator evaluates the generated images to ensure they are realistic. This process helps to upscale low-resolution videos by generating additional pixels and details to improve the overall quality.
Another commonly used neural network for upscaling videos is the Super-Resolution Convolutional Neural Network (SRCNN). SRCNN uses deep learning techniques to enhance image resolution by learning the mapping between low-resolution and high-resolution images.
By training on a dataset of low and high-resolution video frames, SRCNN can effectively upscale videos while preserving important details and reducing noise. Overall, neural networks play a crucial role in upscaling videos by utilizing advanced algorithms to enhance image quality and resolution.
What is the role of gans in video upscaling?
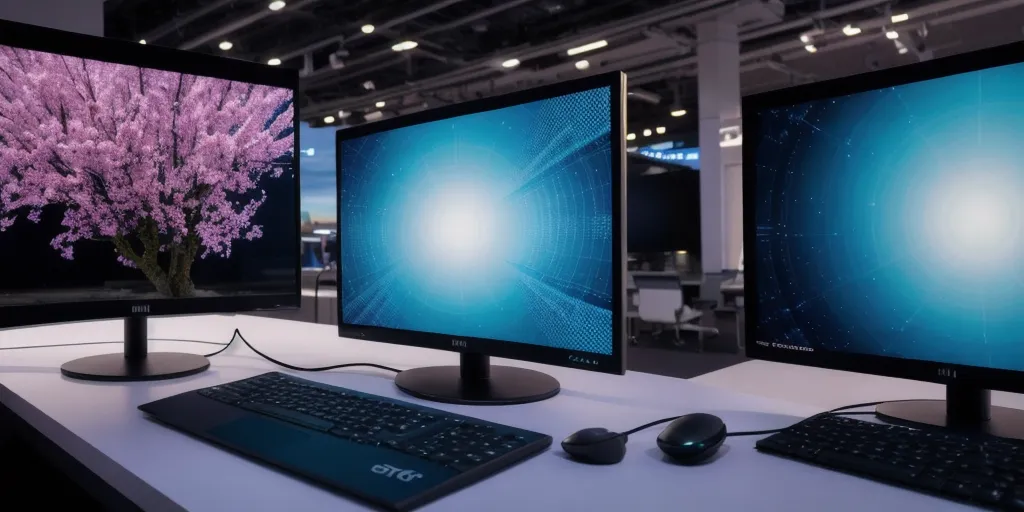
Generative Adversarial Networks (GANs) play a crucial role in video upscaling by utilizing a two-part system consisting of a generator and a discriminator. The generator is responsible for creating high-resolution images from low-resolution inputs, while the discriminator evaluates the generated images to ensure they are realistic and indistinguishable from true high-resolution images.
This process of generating and evaluating images continues iteratively until the generator produces high-quality upscaled videos. GANs are particularly effective in video upscaling as they can capture complex patterns and details in the images, resulting in sharper and more realistic outputs.
Additionally, GANs can learn from a large dataset of images to improve the quality of the upscaled videos over time.
By leveraging the power of GANs, video upscaling techniques can enhance the visual quality of low-resolution videos, making them suitable for high-definition displays and improving the overall viewing experience for audiences. In conclusion, GANs play a vital role in video upscaling by generating high-quality images and improving the visual fidelity of low-resolution videos.
How do ai-powered tools improve video resolution?
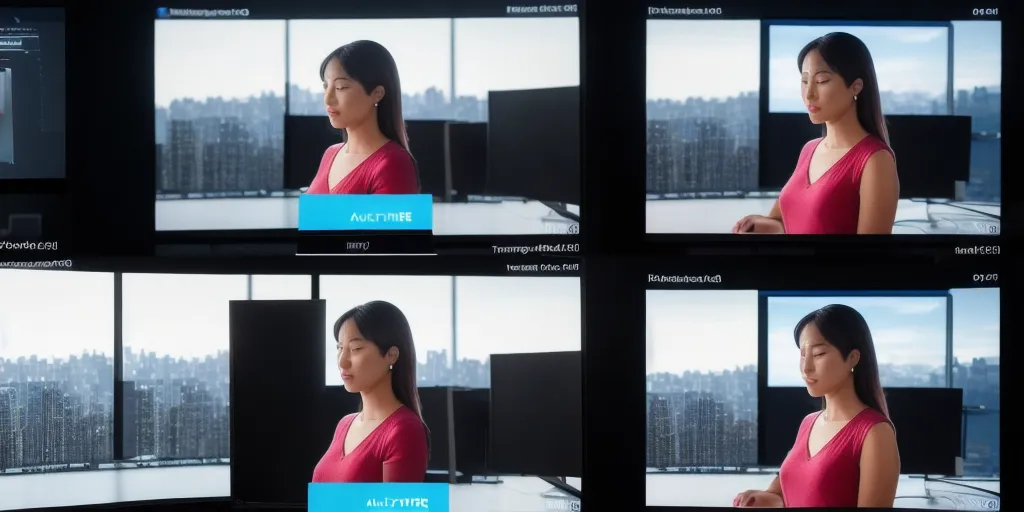
AI-powered tools improve video resolution by utilizing advanced algorithms to enhance the quality of the video content. These tools can analyze the video frame by frame, identifying areas that are blurry or pixelated, and then applying techniques such as image upscaling, noise reduction, and super-resolution to improve the overall clarity and sharpness of the video.
By using machine learning and deep learning algorithms, AI-powered tools can learn from a vast amount of data to continuously improve their ability to enhance video resolution.
Additionally, these tools can also adjust the brightness, contrast, and color balance of the video to create a more visually appealing and professional-looking result. Overall, AI-powered tools provide a cost-effective and efficient solution for improving video resolution, allowing content creators, filmmakers, and video editors to produce high-quality videos without the need for expensive equipment or extensive manual editing.
With the advancements in AI technology, the future of video resolution enhancement looks promising, offering endless possibilities for creating stunning visual content.