AI-generated videos are created using a process known as deep learning, which involves training artificial intelligence algorithms on vast amounts of data. These algorithms are fed with various inputs, such as images, videos, and audio, to learn patterns and generate new content.
One common technique used in generating videos is called Generative Adversarial Networks (GANs), where two neural networks, a generator, and a discriminator, work together to produce realistic videos. The generator creates new video content, while the discriminator evaluates the authenticity of the generated videos.
Through this iterative process, the generator improves its output to create more realistic and convincing videos. Additionally, AI-generated videos can also be created using techniques like style transfer, where the style of one video is applied to another, or by using pre-trained models that can generate videos based on specific inputs.
Overall, AI-generated videos are made by leveraging advanced algorithms and deep learning techniques to produce visually compelling and realistic content.
What is the process for creating ai-generated videos?
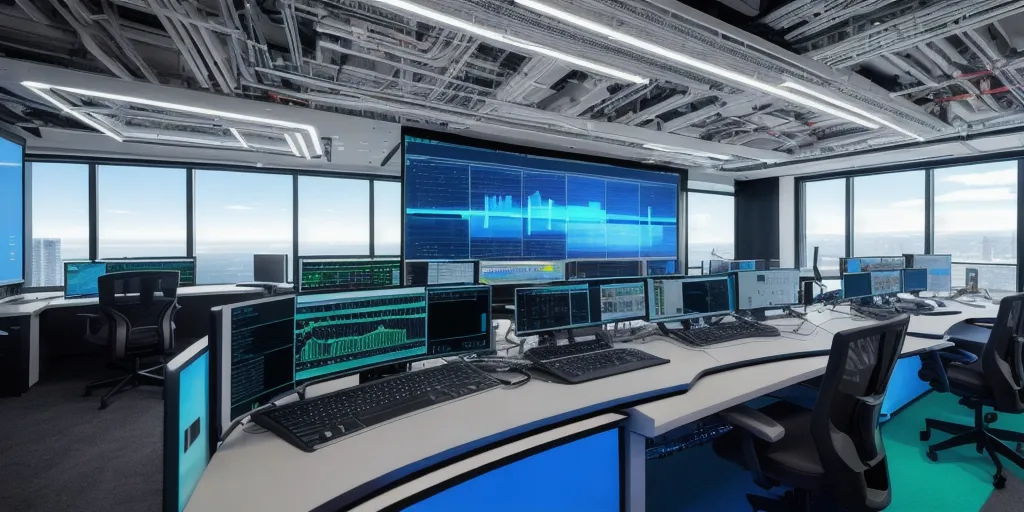
The process for creating AI-generated videos involves several key steps. First, the AI algorithm must be trained using a large dataset of videos to learn patterns and features that can be used to generate new content. This training process requires a significant amount of computational power and time to ensure the AI model is accurate and effective.
Once the AI model is trained, it can then be used to generate new videos by inputting text, images, or other data that the algorithm can use to create a video. The AI model will then use its learned patterns and features to generate a video that aligns with the input data.
After the video is generated, it can be further edited and refined by human editors to ensure quality and coherence. This process of training, generating, and editing AI-generated videos allows for the creation of unique and engaging content that can be used for a variety of purposes, from marketing and advertising to entertainment and education.
Overall, the process for creating AI-generated videos is complex but can result in high-quality and innovative content.
How do deep learning algorithms contribute to making ai-generated videos?
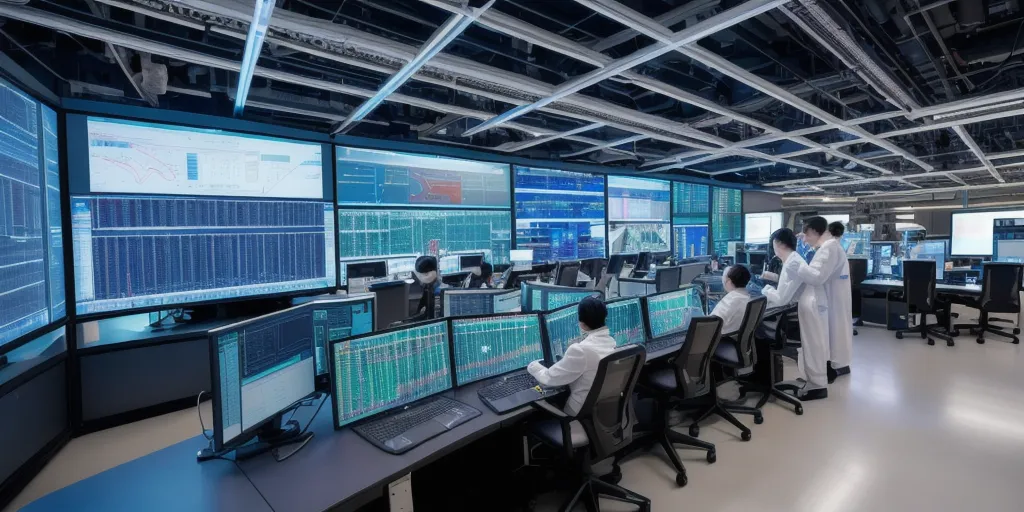
Deep learning algorithms play a crucial role in the creation of AI-generated videos by enabling machines to analyze and understand complex patterns within visual data. These algorithms, which are a subset of machine learning techniques, use neural networks to process vast amounts of information and learn from it to make predictions or decisions.
In the context of video generation, deep learning algorithms can be trained on large datasets of videos to recognize patterns, objects, and movements, allowing them to generate realistic and coherent video content.
By leveraging techniques such as convolutional neural networks (CNNs) and recurrent neural networks (RNNs), deep learning algorithms can capture intricate details and nuances in video data, leading to more accurate and lifelike video outputs. Additionally, deep learning algorithms can also be used to enhance the quality of AI-generated videos by improving aspects such as image resolution, color accuracy, and motion smoothness.
Overall, deep learning algorithms significantly contribute to the advancement of AI-generated videos by enabling machines to process and interpret visual information in a way that closely mimics human perception and creativity.
What role do generative adversarial networks play in generating ai videos?
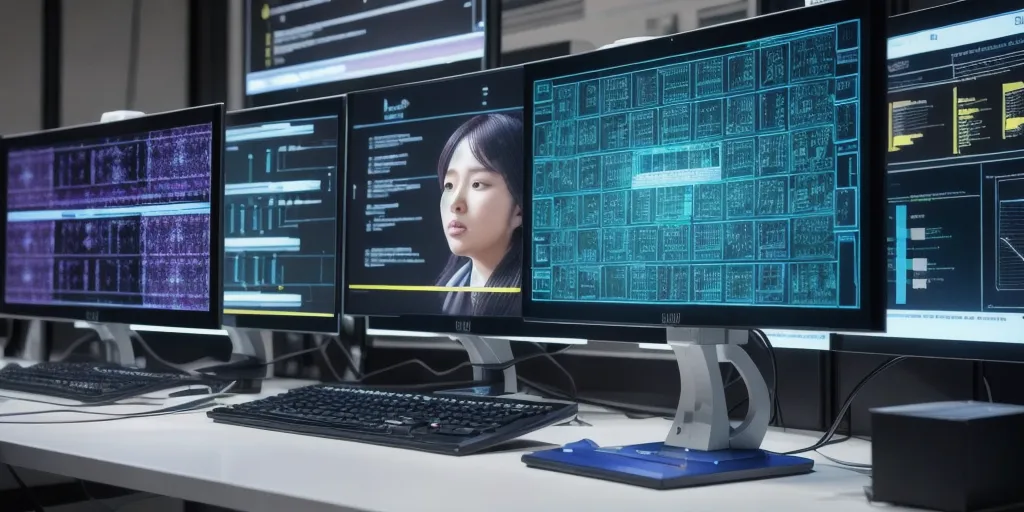
Generative Adversarial Networks (GANs) play a crucial role in generating AI videos by utilizing a unique framework that consists of two neural networks: the generator and the discriminator. The generator is responsible for creating realistic videos by generating new data samples, while the discriminator evaluates these samples to distinguish between real and generated videos.
Through a process of competition and collaboration, GANs are able to improve the quality and realism of the generated videos over time. This iterative process allows GANs to learn from their mistakes and continuously refine their output, resulting in more convincing and lifelike videos.
Additionally, GANs can be trained on a diverse range of video data, enabling them to generate videos in various styles and genres.
By harnessing the power of GANs, researchers and developers can create AI videos that are indistinguishable from real footage, opening up new possibilities for applications in entertainment, education, and beyond. In conclusion, GANs are a powerful tool in the field of AI video generation, offering a sophisticated and effective approach to creating realistic and compelling visual content.
How can style transfer be used to create ai-generated videos?
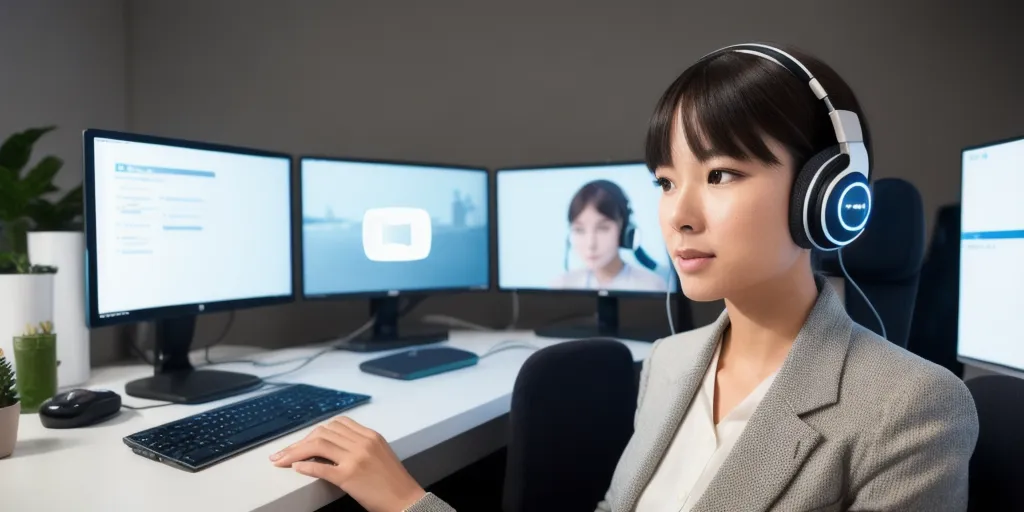
Style transfer can be used to create AI-generated videos by applying the technique of transferring the visual style of one image or video onto another. This process involves using deep learning algorithms to analyze the style of a reference image or video and then applying that style to a target video.
By utilizing neural networks, AI can learn the characteristics of the reference style and apply them to the target video frame by frame, resulting in a visually appealing and cohesive final product. This technology allows for the creation of unique and artistic videos that combine different visual styles and aesthetics.
Additionally, style transfer can be used to enhance the overall quality and creativity of videos by adding artistic elements and transforming mundane footage into visually stunning content.
Overall, style transfer in AI-generated videos offers a powerful tool for content creators to experiment with different styles, create engaging visuals, and push the boundaries of traditional video production techniques.
What techniques are utilized to train ai models for generating videos?
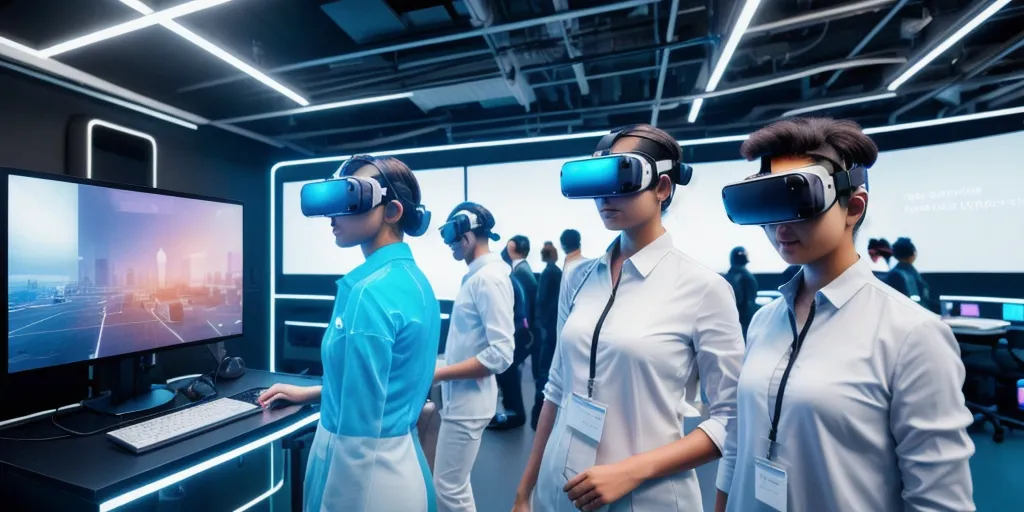
Training AI models for generating videos involves a variety of techniques to ensure optimal performance and accuracy. One common technique is supervised learning, where the AI model is trained on a large dataset of labeled videos to learn patterns and features.
This allows the model to make predictions and generate new videos based on the patterns it has learned. Another technique is reinforcement learning, where the model is rewarded for making correct predictions and generating high-quality videos. This helps the model improve over time through trial and error.
Additionally, techniques such as transfer learning can be utilized to leverage pre-trained models and adapt them to the specific task of video generation. This can help speed up the training process and improve the overall performance of the AI model.
Overall, a combination of these techniques, along with data augmentation and regularization methods, can be used to train AI models effectively for generating videos with high quality and realism. By utilizing these techniques, AI models can learn to generate videos that are indistinguishable from those created by humans, opening up a wide range of possibilities for applications in various industries.